Association analysis between risk factors and cardiovascular age-delta
Contents
Association analysis between risk factors and cardiovascular age-delta#
{contents}
Introduction#
This .rmd uses outputs generated by the first stage of the method. This first stage generates a predicted age that is corrected for ‘regression to the mean’ bias associated in predicting biological age from chronological age.
Inputs are healthy validation set predicted ages and remaining approx. 34K subjects’ predicted ages in the UK Biobank.
Disease 1: Diabetes#
(i) propensity match samples#
Healthy sample is matched to disease sample by age and sex.
First, examine if there are any differences in covariates:
Welch Two Sample t-test
data: age_at_MRI by rf_diabetes
t = -7.2518, df = 4634.4, p-value = 4.795e-13
alternative hypothesis: true difference in means between group 0 and group 1 is not equal to 0
95 percent confidence interval:
-1.1962527 -0.6871013
sample estimates:
mean in group 0 mean in group 1
63.90242 64.84410
Welch Two Sample t-test
data: sex by rf_diabetes
t = -7.1905, df = 4605.6, p-value = 7.497e-13
alternative hypothesis: true difference in means between group 0 and group 1 is not equal to 0
95 percent confidence interval:
-0.07960591 -0.04549683
sample estimates:
mean in group 0 mean in group 1
0.4874623 0.5500136
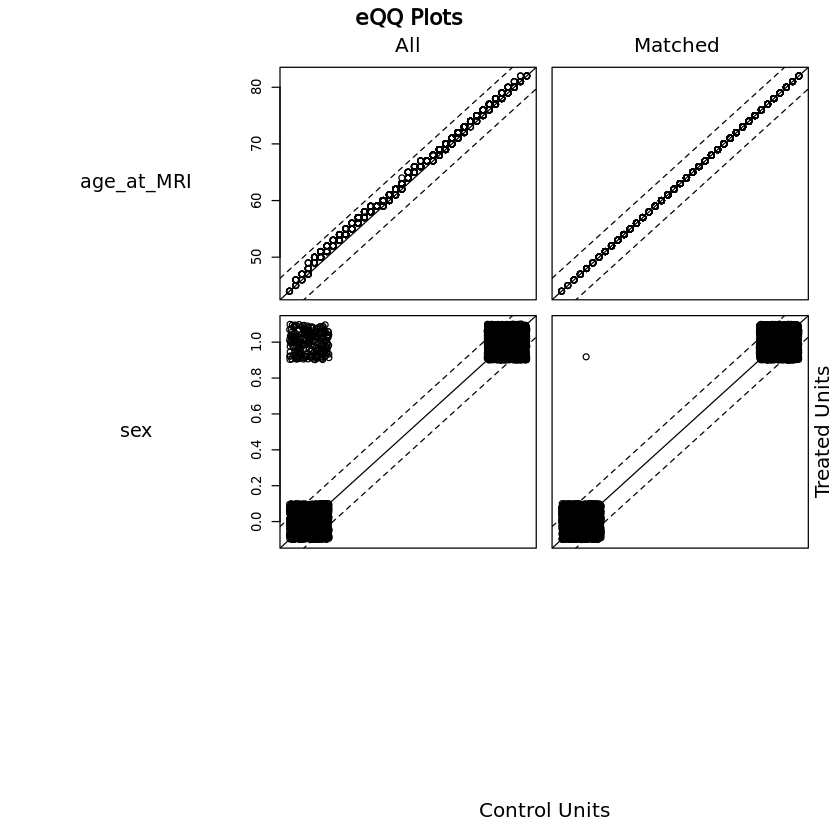
Dimensions of matched data (equal numbers in both groups once matched, number represents total of healthy + disease):
- 7338
- 136
Now compare distributions of matched data:
Warning message:
“`funs()` was deprecated in dplyr 0.8.0.
Please use a list of either functions or lambdas:
# Simple named list:
list(mean = mean, median = median)
# Auto named with `tibble::lst()`:
tibble::lst(mean, median)
# Using lambdas
list(~ mean(., trim = .2), ~ median(., na.rm = TRUE))
This warning is displayed once every 8 hours.
Call `lifecycle::last_lifecycle_warnings()` to see where this warning was generated.”
rf_diabetes | sex |
---|---|
<dbl> | <dbl> |
0 | 0.5500136 |
1 | 0.5500136 |
(ii) analysis#
Summary:#
rf_diabetes | meanca_delta | n |
---|---|---|
<dbl> | <chr> | <int> |
0 | -0.135 | 3669 |
1 | 0.174 | 3669 |
Plot:#
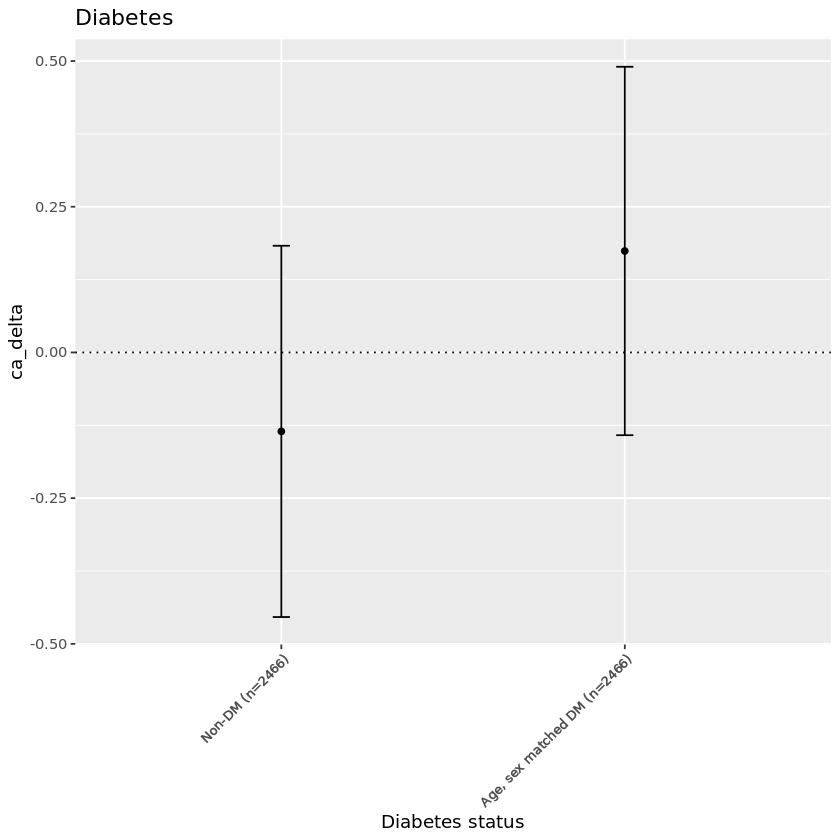
Regression (adjusted for age, age^2, sex):#
Call:
lm(formula = ca_delta ~ rf_diabetes + poly(age_at_MRI, 2) + sex,
data = dta_m)
Residuals:
Min 1Q Median 3Q Max
-33.248 -6.850 -0.218 6.791 34.131
Coefficients:
Estimate Std. Error t value Pr(>|t|)
(Intercept) 0.1550 0.2055 0.754 0.4507
rf_diabetes 0.3096 0.2289 1.353 0.1762
poly(age_at_MRI, 2)1 -4.3951 9.8172 -0.448 0.6544
poly(age_at_MRI, 2)2 11.8102 9.8023 1.205 0.2283
sex -0.5282 0.2304 -2.293 0.0219 *
---
Signif. codes: 0 ‘***’ 0.001 ‘**’ 0.01 ‘*’ 0.05 ‘.’ 0.1 ‘ ’ 1
Residual standard error: 9.802 on 7333 degrees of freedom
Multiple R-squared: 0.001205, Adjusted R-squared: 0.0006602
F-statistic: 2.212 on 4 and 7333 DF, p-value: 0.06514
2.5 % | 97.5 % | |
---|---|---|
(Intercept) | -0.2478601 | 0.55792884 |
rf_diabetes | -0.1390727 | 0.75818057 |
poly(age_at_MRI, 2)1 | -23.6397501 | 14.84949413 |
poly(age_at_MRI, 2)2 | -7.4051133 | 31.02549636 |
sex | -0.9797560 | -0.07659024 |
Call:
lm(formula = ca_delta ~ poly(age_at_MRI, 2) + sex * rf_diabetes,
data = dta_m)
Residuals:
Min 1Q Median 3Q Max
-33.316 -6.844 -0.200 6.812 34.063
Coefficients:
Estimate Std. Error t value Pr(>|t|)
(Intercept) 0.08751 0.24135 0.363 0.717
poly(age_at_MRI, 2)1 -4.39513 9.81772 -0.448 0.654
poly(age_at_MRI, 2)2 11.81019 9.80277 1.205 0.228
sex -0.40541 0.32555 -1.245 0.213
rf_diabetes 0.44460 0.34118 1.303 0.193
sex:rf_diabetes -0.24553 0.46004 -0.534 0.594
Residual standard error: 9.803 on 7332 degrees of freedom
Multiple R-squared: 0.001244, Adjusted R-squared: 0.0005628
F-statistic: 1.826 on 5 and 7332 DF, p-value: 0.1041
2.5 % | 97.5 % | |
---|---|---|
(Intercept) | -0.3856115 | 0.5606381 |
poly(age_at_MRI, 2)1 | -23.6406891 | 14.8504331 |
poly(age_at_MRI, 2)2 | -7.4060508 | 31.0264339 |
sex | -1.0435851 | 0.2327641 |
rf_diabetes | -0.2242198 | 1.1134120 |
sex:rf_diabetes | -1.1473452 | 0.6562948 |
Call:
lm(formula = ca_delta ~ poly(age_at_MRI, 2) + I(1 - sex) * rf_diabetes,
data = dta_m)
Residuals:
Min 1Q Median 3Q Max
-33.316 -6.844 -0.200 6.812 34.063
Coefficients:
Estimate Std. Error t value Pr(>|t|)
(Intercept) -0.3179 0.2183 -1.456 0.145
poly(age_at_MRI, 2)1 -4.3951 9.8177 -0.448 0.654
poly(age_at_MRI, 2)2 11.8102 9.8028 1.205 0.228
I(1 - sex) 0.4054 0.3256 1.245 0.213
rf_diabetes 0.1991 0.3086 0.645 0.519
I(1 - sex):rf_diabetes 0.2455 0.4600 0.534 0.594
Residual standard error: 9.803 on 7332 degrees of freedom
Multiple R-squared: 0.001244, Adjusted R-squared: 0.0005628
F-statistic: 1.826 on 5 and 7332 DF, p-value: 0.1041
2.5 % | 97.5 % | |
---|---|---|
(Intercept) | -0.7458100 | 0.1100155 |
poly(age_at_MRI, 2)1 | -23.6406891 | 14.8504331 |
poly(age_at_MRI, 2)2 | -7.4060508 | 31.0264339 |
I(1 - sex) | -0.2327641 | 1.0435851 |
rf_diabetes | -0.4058792 | 0.8040210 |
I(1 - sex):rf_diabetes | -0.6562948 | 1.1473452 |
Disease 2: Hypertension#
(i) propensity match samples#
Healthy sample is matched to disease sample by age and sex.
First, examine if there are any differences in covariates.
Welch Two Sample t-test
data: age_at_MRI by rf_htn
t = -27.257, df = 32186, p-value < 2.2e-16
alternative hypothesis: true difference in means between group 0 and group 1 is not equal to 0
95 percent confidence interval:
-2.376973 -2.058054
sample estimates:
mean in group 0 mean in group 1
63.03944 65.25696
Welch Two Sample t-test
data: sex by rf_htn
t = -13.35, df = 31921, p-value < 2.2e-16
alternative hypothesis: true difference in means between group 0 and group 1 is not equal to 0
95 percent confidence interval:
-0.08336211 -0.06201811
sample estimates:
mean in group 0 mean in group 1
0.4625790 0.5352692
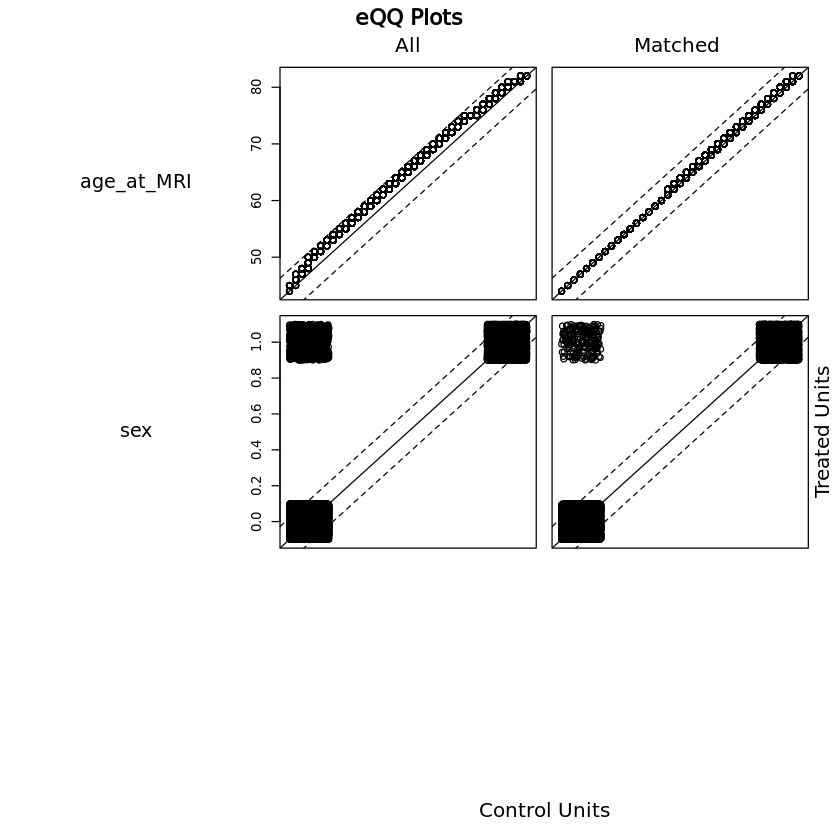
Dimensions of matched data (equal numbers in both groups once matched, number represents total of healthy + htn)
- 29686
- 137
Now compare distributions of matched data
rf_htn | sex |
---|---|
<dbl> | <dbl> |
0 | 0.5226706 |
1 | 0.5352692 |
(ii) analysis#
Summary:#
rf_htn | mean_ca_delta | n |
---|---|---|
<dbl> | <chr> | <int> |
0 | -0.748 | 14843 |
1 | 0.821 | 14843 |
Plot:#
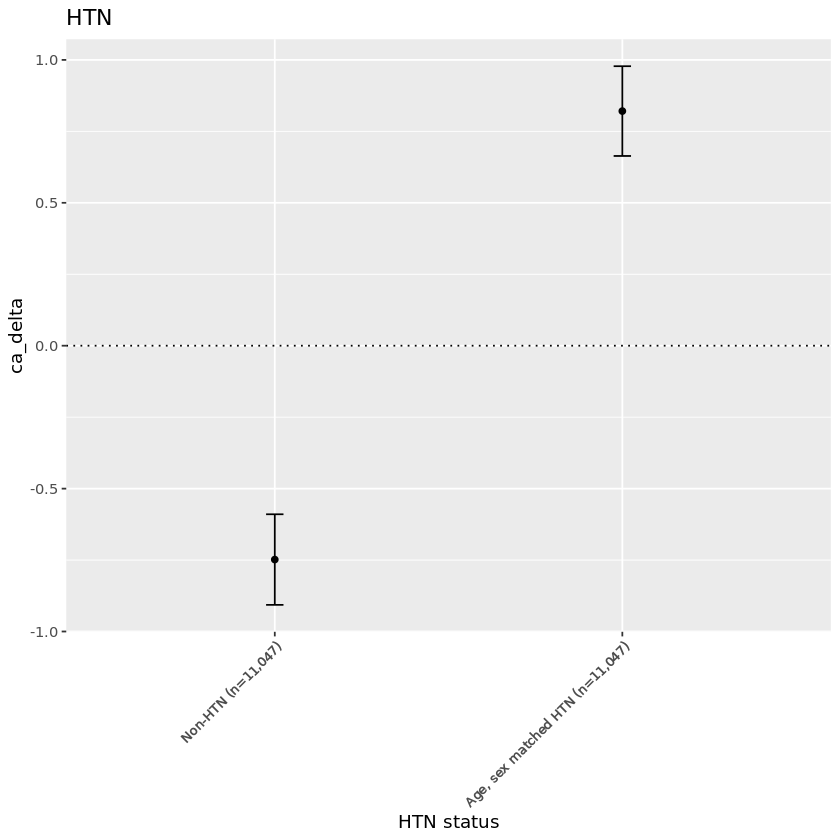
Regression (adjusted for age, age^2, sex):#
Call:
lm(formula = ca_delta ~ rf_htn + age_at_MRI + poly(age_at_MRI,
2) + sex, data = dta_m_htn)
Residuals:
Min 1Q Median 3Q Max
-36.609 -6.815 -0.133 6.809 34.599
Coefficients: (1 not defined because of singularities)
Estimate Std. Error t value Pr(>|t|)
(Intercept) 0.074600 0.517591 0.144 0.885
rf_htn 1.571240 0.113906 13.794 < 2e-16 ***
age_at_MRI -0.007320 0.007857 -0.932 0.352
poly(age_at_MRI, 2)1 NA NA NA NA
poly(age_at_MRI, 2)2 22.142638 9.809253 2.257 0.024 *
sex -0.657250 0.114046 -5.763 8.34e-09 ***
---
Signif. codes: 0 ‘***’ 0.001 ‘**’ 0.01 ‘*’ 0.05 ‘.’ 0.1 ‘ ’ 1
Residual standard error: 9.802 on 29681 degrees of freedom
Multiple R-squared: 0.007692, Adjusted R-squared: 0.007559
F-statistic: 57.52 on 4 and 29681 DF, p-value: < 2.2e-16
2.5 % | 97.5 % | |
---|---|---|
(Intercept) | -0.93990066 | 1.089100481 |
rf_htn | 1.34797958 | 1.794500451 |
age_at_MRI | -0.02272063 | 0.008080425 |
poly(age_at_MRI, 2)1 | NA | NA |
poly(age_at_MRI, 2)2 | 2.91607216 | 41.369203679 |
sex | -0.88078623 | -0.433714697 |
Call:
lm(formula = ca_delta ~ poly(age_at_MRI, 2) + sex * rf_htn, data = dta_m_htn)
Residuals:
Min 1Q Median 3Q Max
-36.587 -6.825 -0.133 6.808 34.579
Coefficients:
Estimate Std. Error t value Pr(>|t|)
(Intercept) -0.42371 0.11647 -3.638 0.000275 ***
poly(age_at_MRI, 2)1 -9.03378 9.81466 -0.920 0.357352
poly(age_at_MRI, 2)2 22.21658 9.81141 2.264 0.023559 *
sex -0.61495 0.16113 -3.817 0.000136 ***
rf_htn 1.61602 0.16581 9.746 < 2e-16 ***
sex:rf_htn -0.08477 0.22811 -0.372 0.710169
---
Signif. codes: 0 ‘***’ 0.001 ‘**’ 0.01 ‘*’ 0.05 ‘.’ 0.1 ‘ ’ 1
Residual standard error: 9.802 on 29680 degrees of freedom
Multiple R-squared: 0.007697, Adjusted R-squared: 0.00753
F-statistic: 46.04 on 5 and 29680 DF, p-value: < 2.2e-16
2.5 % | 97.5 % | |
---|---|---|
(Intercept) | -0.6519931 | -0.1954327 |
poly(age_at_MRI, 2)1 | -28.2709396 | 10.2033823 |
poly(age_at_MRI, 2)2 | 2.9857783 | 41.4473769 |
sex | -0.9307677 | -0.2991345 |
rf_htn | 1.2910183 | 1.9410220 |
sex:rf_htn | -0.5318870 | 0.3623382 |
Call:
lm(formula = ca_delta ~ poly(age_at_MRI, 2) + sex * rf_htn, data = dta_m_htn)
Residuals:
Min 1Q Median 3Q Max
-36.587 -6.825 -0.133 6.808 34.579
Coefficients:
Estimate Std. Error t value Pr(>|t|)
(Intercept) -0.42371 0.11647 -3.638 0.000275 ***
poly(age_at_MRI, 2)1 -9.03378 9.81466 -0.920 0.357352
poly(age_at_MRI, 2)2 22.21658 9.81141 2.264 0.023559 *
sex -0.61495 0.16113 -3.817 0.000136 ***
rf_htn 1.61602 0.16581 9.746 < 2e-16 ***
sex:rf_htn -0.08477 0.22811 -0.372 0.710169
---
Signif. codes: 0 ‘***’ 0.001 ‘**’ 0.01 ‘*’ 0.05 ‘.’ 0.1 ‘ ’ 1
Residual standard error: 9.802 on 29680 degrees of freedom
Multiple R-squared: 0.007697, Adjusted R-squared: 0.00753
F-statistic: 46.04 on 5 and 29680 DF, p-value: < 2.2e-16
2.5 % | 97.5 % | |
---|---|---|
(Intercept) | -0.6519931 | -0.1954327 |
poly(age_at_MRI, 2)1 | -28.2709396 | 10.2033823 |
poly(age_at_MRI, 2)2 | 2.9857783 | 41.4473769 |
sex | -0.9307677 | -0.2991345 |
rf_htn | 1.2910183 | 1.9410220 |
sex:rf_htn | -0.5318870 | 0.3623382 |
Forest plot: Categorical variables#
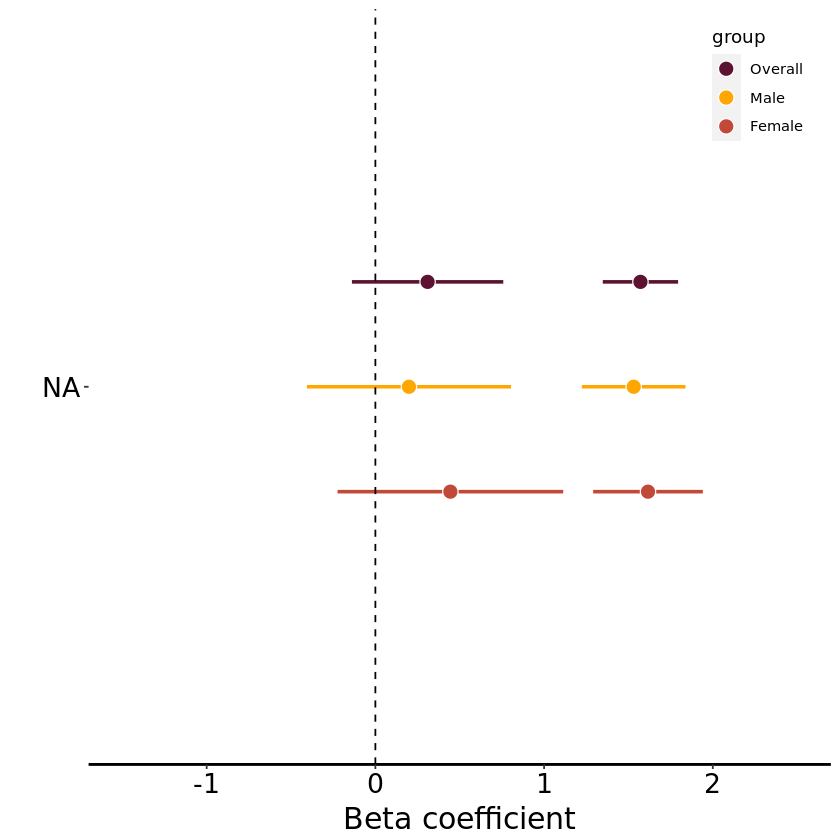